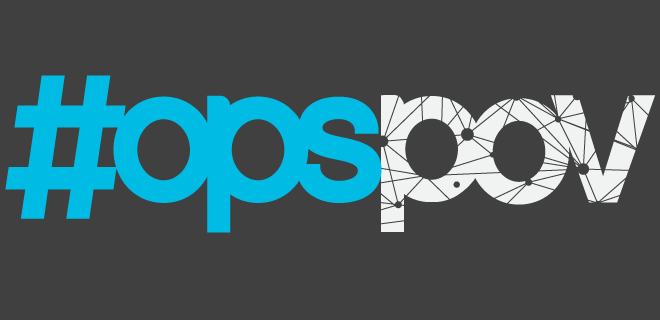
With vague regulations popping up that seem impossibly to comply with as browsers commit to a crackdown on third-party cookies, you’re probably wondering what’s in store for audience-based ad targeting. Then you notice your old friend Peer39 was just spun out of Sizmek and a voice in the back of your head wonders: “Wait—does this mean semantic and contextual targeting is coming back big time?”
Now it’s all right to feel once bitten and twice shy when talking about the revival of contextual targeting—this isn’t the first time that theory has spun around the ad-tech rumor mill only to fizzle out in less-than-glorious fashion. However, in prepping for our PubForum Vancouver panel—”How Will Enhanced User Privacy Reshape Programmatic“—Smart EVP of Americas Joe Lospalluto and I got into an in-depth discussion about the many reasons why this contextual comeback is the real deal.
GAVIN DUNAWAY: Why is this the right time for semantic targeting to make a comeback?
JOE LOSPALLUTO: Privacy and regulatory concerns have changed the way publishers and advertisers are approaching audience targeting. These concerns are at the heart of an ongoing challenge for advertisers to reach the scale they need without cookies. The amount of available behavioral data is shrinking on the open web and the ecosystem needs to find valuable alternatives.
Given the changes to the Safari browser and those coming to the Chrome and Firefox browsers, it’s clear the market needs to prepare for the post-cookie era. Semantic targeting isn’t the entire solution to these issues, but it can certainly play an important role.
There is an immediate risk to most publishers that are not Google or Facebook. DTC and other performance advertisers have already flocked to these big walled gardens—not because they love the contextual environment, but because the data is so rich and it drives performance.
To the degree that these advertisers still buy outside of the walled gardens, this spend will likely drop considerably without the benefit of audience targeting. SSPs and publishers already see significant decreases in the revenue generated from audiences on the Safari browser. It is likely this will also be the case with Chrome over time.
GD: How does semantic targeting address advertisers’ needs?
JL: Semantic contextual targeting is all about displaying ads that are relevant to the editorial context in which they’re placed. This match between the ad and the content of the viewed page optimizes the user’s stimulated and heightened attention towards the ad because it aligns with the content subject matter. In other words, it’s the right content, right place, right time. That means increased ad-performance and user engagement.
This association between the ad and the content will positively impact the consumer’s perception and therefore ensure brand safety. This is particularly valuable for brand advertisers where the publisher’s brand and high-quality environment have a halo effect on the perception of the brand as there will be greater alignment with the brand identity, values and strategy over time.
Brand advertisers have long understood the value of context and that understanding is still clearly reflected in their buying in non-digital channels. Audience buying in programmatic has largely ignored context except at the most rudimentary levels.
To the degree... advertisers still buy outside of the walled gardens, this spend will likely drop considerably without the benefit of audience targeting. SSPs and publishers already see significant decreases in the revenue generated from audiences on the Safari browser.
GD: Why does semantic targeting address publishers’ needs?
JL: The (re-)adoption of semantic contextual targeting is one way that publishers can leverage the unique value of their brands, their audiences, and their content. This, of course, benefits the buyer as well as the entire value chain in a shared interest business approach. Context/content is one type of publishers’ first-party data and audience data is the another.
Certainly, semantic targeting alone is not always the solution. The most powerful combination is when contextual signals are used in combination with publishers’ audience data. This allows publishers to fully understand their content, their audience, and how they interact. We’re already helping our publishers using our semantic tools to create programmatic deals that are very attractive to buyers and the response and results have been strong.
When provided with well-curated audiences and contexts, brand advertisers will see better performance reflected in their brand metrics, which will drive demand for better-qualified inventory. In addition to this, as ads are more relevant to the content, it will benefit the overall user experience.
GD: How is Smart’s approach to semantic data different from ones we’ve seen rise and fall over the years?
JL: Most of the current approaches to semantic data are based on keywords: give a list of keywords corresponding to your semantic segment and the semantic engine will include the pages containing the keywords in your segment. These approaches are not precise at all, they are too dependent upon a precise choice of keywords.
What they fail to do is to take the context in which each word is written into account. Moreover, it can require a lot of time to choose “good-enough” keywords—and you can’t go further than “good-enough.”
Smart’s approach leverages state-of-the-art deep learning techniques that are able to understand the true meaning of the words according to the context in which they appear. Instead of building a list of keywords, we focus on the meaning of each word in its context, which enables us to build models that are much more precise and robust.
GD: Sounds highly technical. Could you give a layman an idea how it works?
JL: Ever-increasing CPU power is making machine learning more powerful every day. This creates a great opportunity for powerful algorithms to do more than ever before. Our semantic engine supports multiple languages representing over 90% of our pages, classifies with hundreds of millions of pages categorized so far.
When a new page is detected in Smart’s inventory, the system downloads and extracts the relevant content and classifies with state-of-the-art natural language processing algorithms. Currently, all IAB Tier 1 categories and 50 IAB Tier 2 categories are handled, as well as major brand safety categories like adult content and hate speech detection. The engine is also able to detect 23 different languages and creates “language” segments. This enables the advertiser to target the language of the content, not only the language of the browser.
For each category handled by our engine, we built a big dataset to train our deep learning algorithms that are able to understand words and sentences in their context. After extracting the meaningful text content of a webpage, the engine applies the models to tag URLs with the appropriate segments.
Most of the current approaches to semantic data are based on keywords: give a list of keywords corresponding to your semantic segment and the semantic engine will include the pages containing the keywords in your segment... What they fail to do is to take the context in which each word is written into account. Moreover, it can require a lot of time to choose “good-enough” keywords—and you can’t go further than “good-enough."
GD: That’s pretty detailed. How do you differentiate your data marketplace from the offerings at other SSPs?
JL: The goal of Smart’s data marketplace is to give full power and control to publishers. Data can be activated on programmatic campaigns and also on direct campaigns in our ad server and this is a strong differentiator as it has always been very difficult to activate data on such campaigns.
The data marketplace already includes the Smart proprietary semantic contextual tools and also several 3rd party audience data providers that cover main geos and platforms to best serve our clients’ needs. Other data points such as proprietary viewability measurement also complete the Smart data marketplace.
Third-party data products carry pricey minimum fees for publishers. We offer these products on a pure CPM basis to our clients. Beyond first-, second- and third-party, we have built a flexible and scalable model with an intuitive workflow that allows any type of publisher to activate the right data, at the right time to best meet advertisers’ needs.
This approach enables Smart to answer smaller publishers’ needs, even those who do not have DMP capabilities. It’s also a true asset for publisher data alliances that can securely control the activation and distribution of their data.
GD: Besides semantic, what do you think are the most interesting areas of development in the data space?
JL: Publishers are our clients. Our job is to help them monetize their unique assets: Publisher brand, content, and audience. Smart is driven by the notion of value path optimization and finding opportunities to bring buyers and sellers closer together to benefit from their shared interests. Semantic contextual targeting will continue to evolve to benefit these two parties.
Contextual targeting, combined with other signals such as location and product data feeds will create new opportunities for both advertisers and publishers. By bringing together contextual targeting and dynamic creative optimization, a brand will be able to adapt its offer and its message to the content in a more refined way. As brands become aware of these capabilities, semantic contextual targeting will become a critical signal in driving performance.
Beyond semantic, we always aim to find new value from the data. We are currently just scratching the surface. By managing billions of auctions per month, every day we collect many terabytes of information about the publisher, the DSP, the web visitor, and much more. These data are the source of exciting areas of development for our data engineering, data science, and data analytics teams.
Smart is a strongly data-driven company and we manage to make every piece of data useful to our day-to-day operations. Our goal is also to optimize and increase the revenues of our customers and the performance, value and technical/operational efficiencies to our partners. This goal is enabled through collecting and analyzing data in depth.