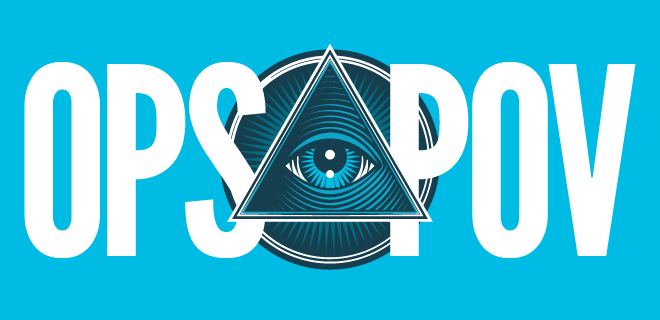
Since Index Exchange’s Andrew Casale explained to me how single-site header architecture could pave the road to fluid ad placements, I’ve been obsessed with the idea.
Instead of a bunch of static spots on a page or within an app where advertisers can shove their wares, what if you had a tabula rasa and could paint the placements and formats that would drive the most revenue per page/session—while similarly improving user experience? All in all, it could make for happier advertisers, users, and publishers.
It’s not as far-fetched as it may sound, which is exactly why I was intrigued by Browsi’s “ad-inventory-as-a-service” and AI-based placements. I caught up with VP of Marketing Alon Even to hear more of the thinking behind these bold programs and how Browsi’s viewability prediction technology opens the door to real-time pricing maneuvers.
GAVIN DUNAWAY: Could you illuminate what “Ad-Inventory-as-a-Service” means to Browsi?
ALON EVEN: Ad-Inventory-as-a-Service is a new category we have defined at Browsi. We’ve noticed a disadvantageous gap between how publishers create, optimize, and price their ad inventory and how advertisers buy their inventory. On the publisher side, this is a manual process mediated by multiple teams (user experience, product, research and development, and business), and that inefficient process repeats itself every few months.
Simply put, when a publisher’s page is loading, no technology (and obviously no human being) is there to give the page’s ad inventory the same level of insight around how to create and sell the inventory, like the advertisers have when buying. This leads to suboptimal supply and revenue loss.
We figured out that unless publishers put a dedicated technology behind their ad inventory, they will never be on even ground with advertisers. The dedicated technology we came up with is Ad-Inventory-as-a-Service.
Browsi enables publishers to regain control over their ad inventory by:
- Automatically creating ad layout per user/per page in real-time that produces the highest combination of viewability, scale, and UX.
- Predicting viewability at the ad-call level, which drives advertisers to bid higher for viewable inventory.
GD: Publishers are always skeptical when the acronym AI appears before a term—why should they instead be intrigued by “AI-based placements”?
AE: You are right, and I understand the skepticism. AI/ML is a buzzword many solutions tend to overuse. However, in our case, it is the core technology behind Browsi’s solution, and we are actually heavy users of Amazon’s AI framework.
Dynamic ad placements is our terminology for “AI-based ad placements” (or fluid ad placements as you mentioned). We believe that only both real-time and historic data can predict the right creation, optimization and pricing of a given ad placement.
If data can help you understand that a given ad placement should be created in a different area on the page than its usual location (or simply eager-loaded instead of the intended lazy loading) to create a viewable impression, then an AI solution can be built for it, and that is exactly what we did.
As part of the AI-based placements, we added all the decisions that are made by human intelligence manually—i.e. the prediction of viewability (today historic data) and pricing of viewability.
GD: What’s key to Browsi’s viewability predictions and how can publishers use that to adjust pricing in real-time?
AE: Using viewability at the ad placement level, which relies on historical data, is simply not enough for publishers. If publishers keep relying on their “usual” high viewable ad placements one of two things will happen: They will miss viewable impressions from lower-performing ad units or they will serve non-viewable impressions in high-performing ad units.
This is why we believe that the chance for viewability needs to be recalculated at the ad call level. Browsi’s viewability prediction enables publishers to automatically allocate the right demand using real-time data to reduce their impression waste. Its value can be translated in the publisher ad server to:
- Guarantee viewability for direct campaigns and avoid impressions waste. For example, send all 70% predicted viewability ad requests to a specific line item and serve all other ad requests to open auction.
- Set the right floor price in the open auction. Programmatic advertisers are looking for viewability and bid more for a higher chance of viewability. Being able to discern, in real-time, clusters of high viewability within a given ad unit, and increase their floors, enables publishers to put price stress on that ad unit.